Identifying common transcriptome signatures of cancer by interpreting deep learning models, Genome Biology
Por um escritor misterioso
Last updated 29 março 2025
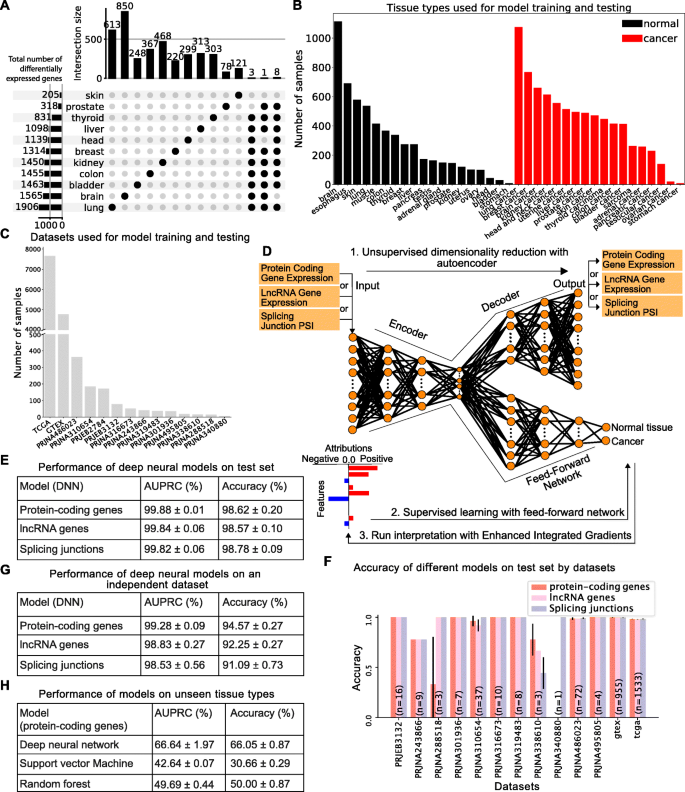
Background Cancer is a set of diseases characterized by unchecked cell proliferation and invasion of surrounding tissues. The many genes that have been genetically associated with cancer or shown to directly contribute to oncogenesis vary widely between tumor types, but common gene signatures that relate to core cancer pathways have also been identified. It is not clear, however, whether there exist additional sets of genes or transcriptomic features that are less well known in cancer biology but that are also commonly deregulated across several cancer types. Results Here, we agnostically identify transcriptomic features that are commonly shared between cancer types using 13,461 RNA-seq samples from 19 normal tissue types and 18 solid tumor types to train three feed-forward neural networks, based either on protein-coding gene expression, lncRNA expression, or splice junction use, to distinguish between normal and tumor samples. All three models recognize transcriptome signatures that are consistent across tumors. Analysis of attribution values extracted from our models reveals that genes that are commonly altered in cancer by expression or splicing variations are under strong evolutionary and selective constraints. Importantly, we find that genes composing our cancer transcriptome signatures are not frequently affected by mutations or genomic alterations and that their functions differ widely from the genes genetically associated with cancer. Conclusions Our results highlighted that deregulation of RNA-processing genes and aberrant splicing are pervasive features on which core cancer pathways might converge across a large array of solid tumor types.

A network medicine approach for identifying diagnostic and
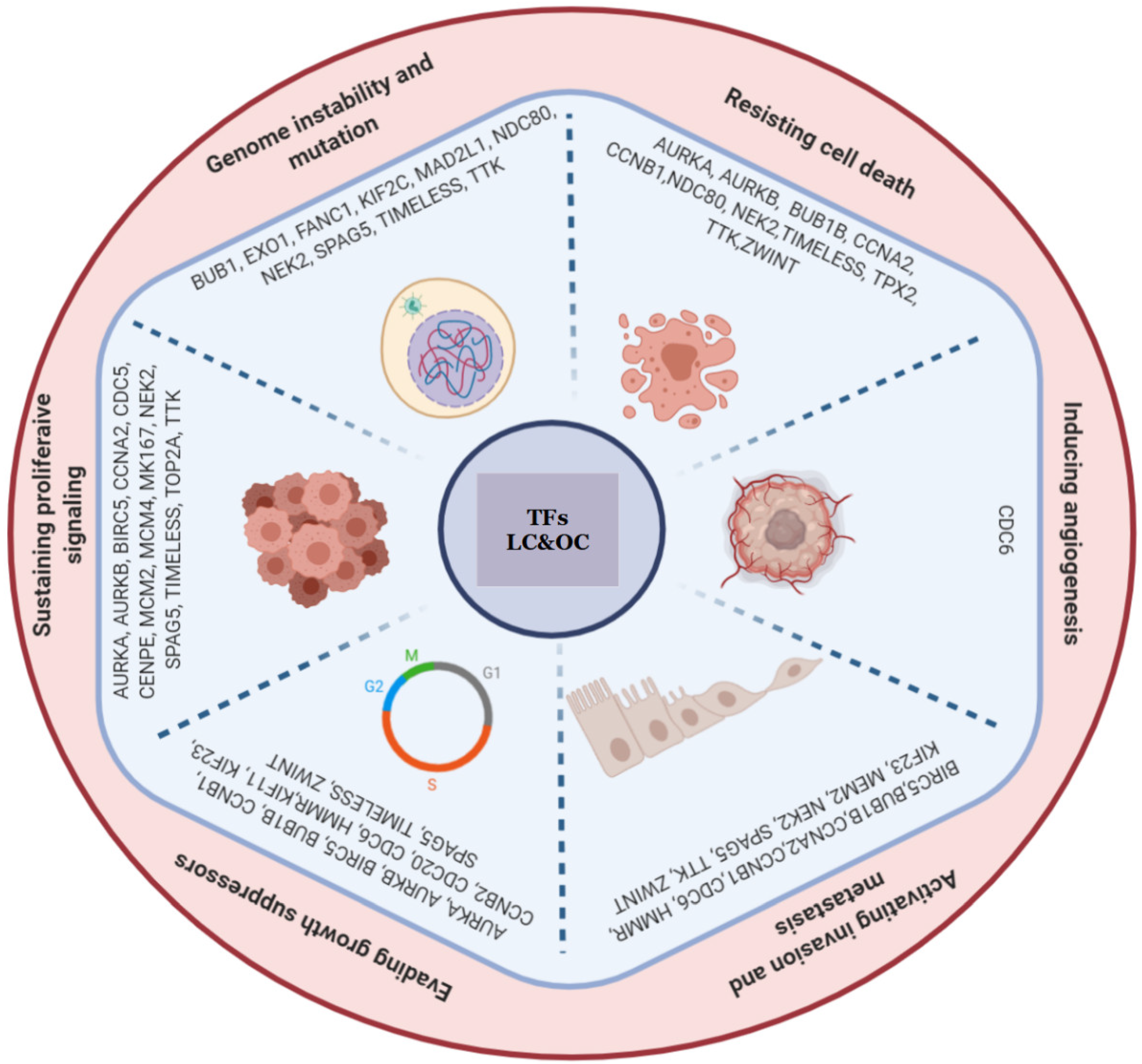
Biology, Free Full-Text
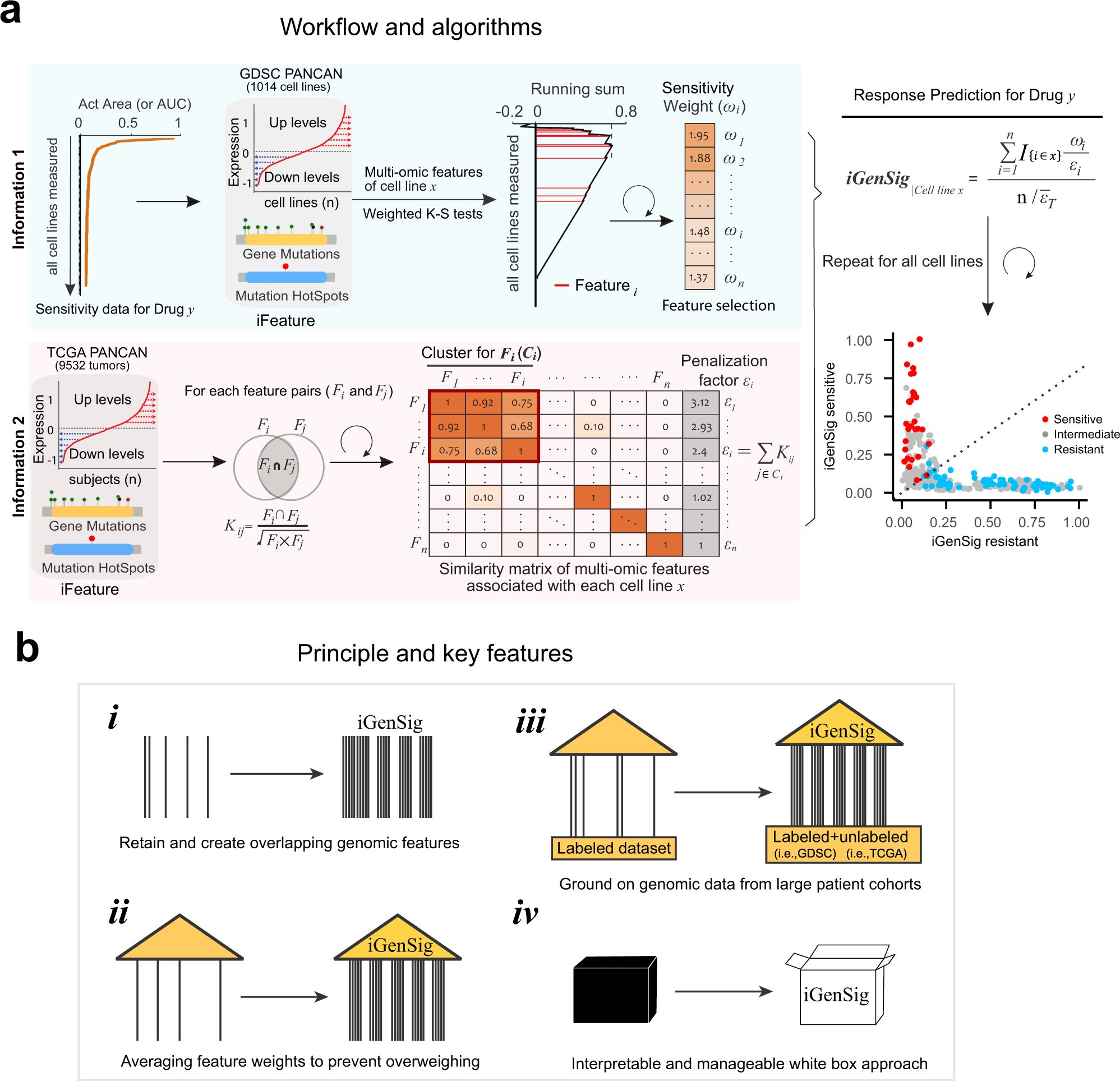
An integral genomic signature approach for tailored cancer therapy
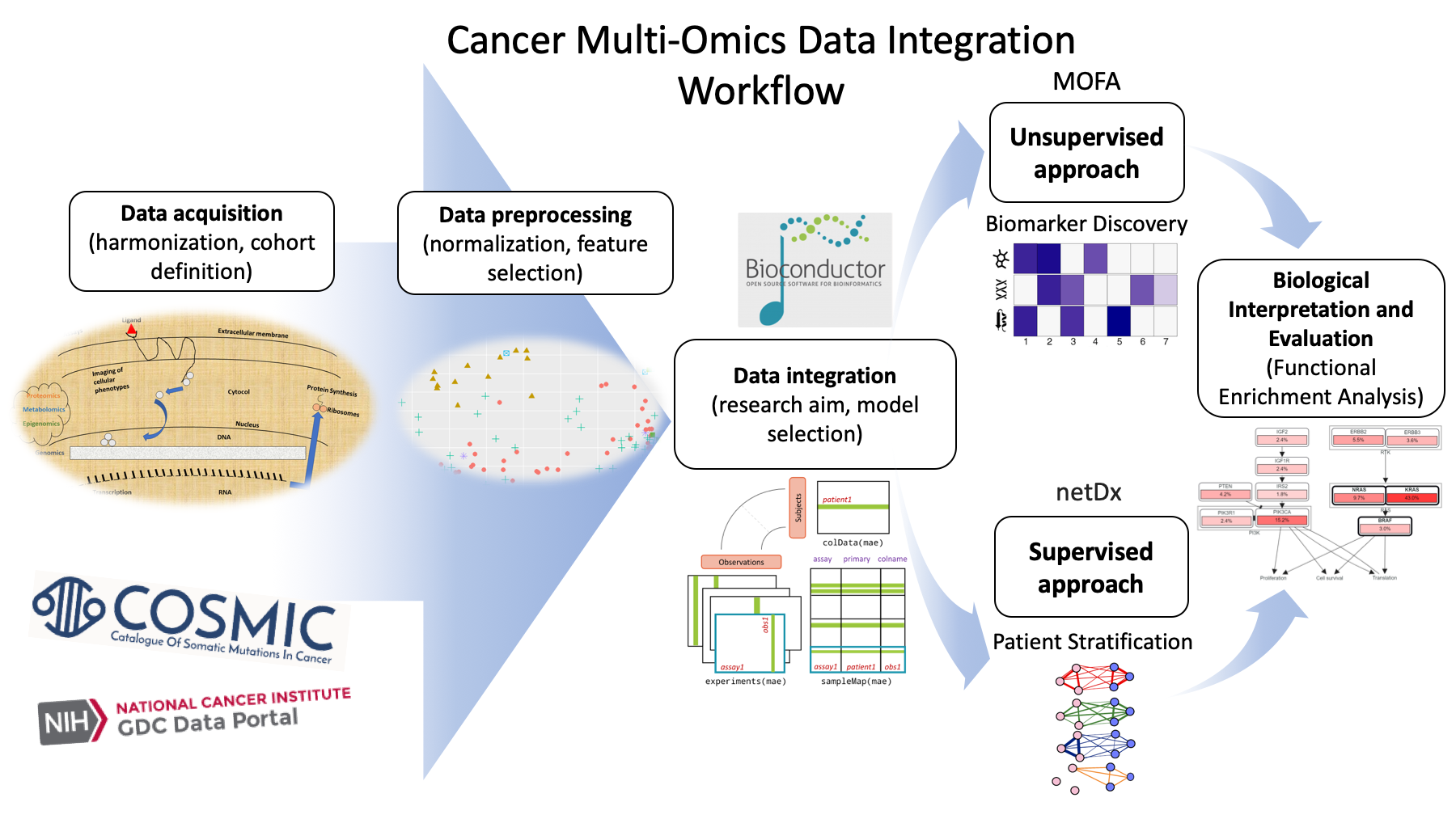
IJMS, Free Full-Text
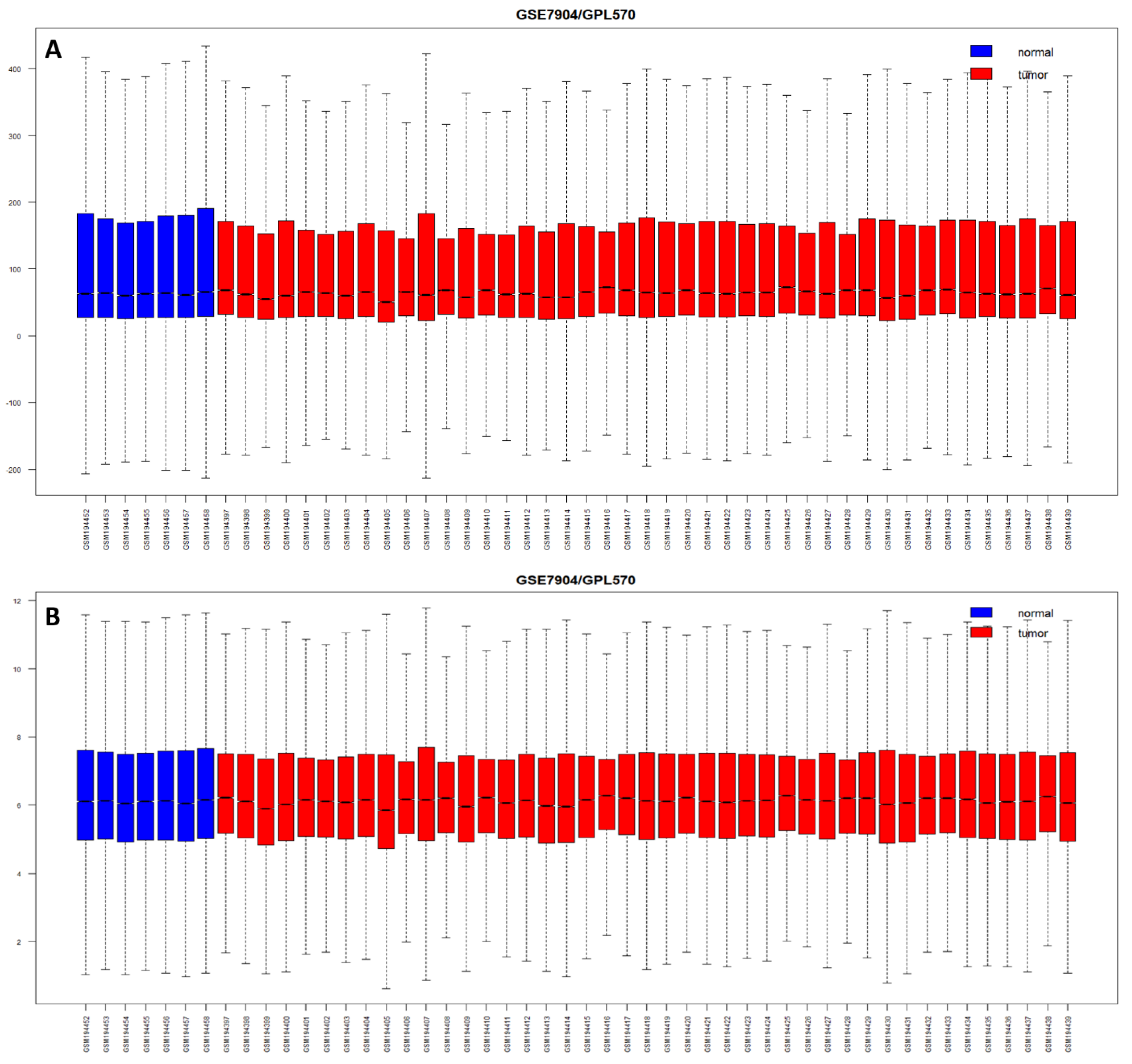
Cancers, Free Full-Text
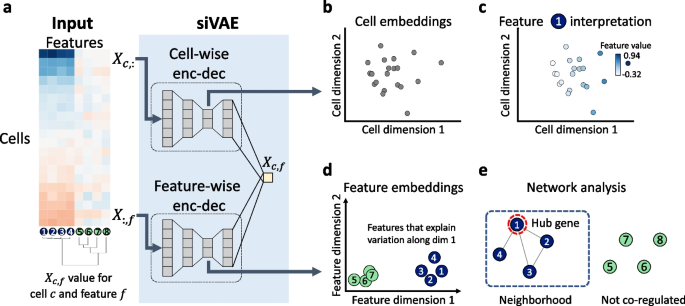
siVAE: interpretable deep generative models for single-cell
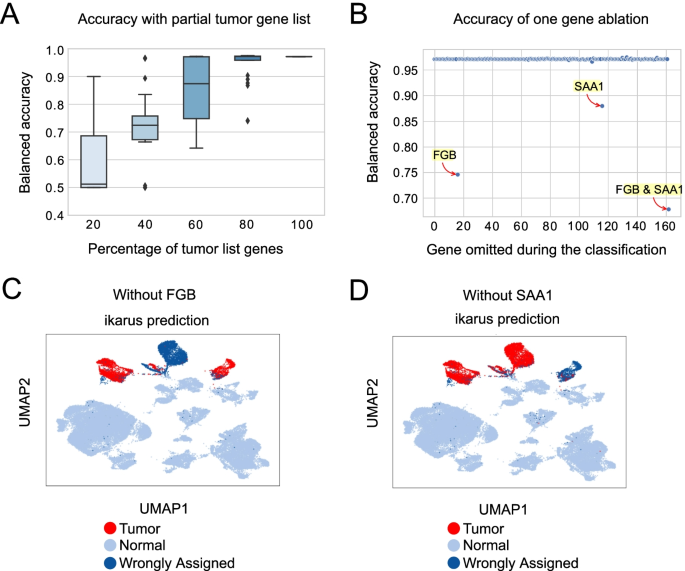
Identifying tumor cells at the single-cell level using machine

Biociphers lab Department of Genetics University of Pennsylvania
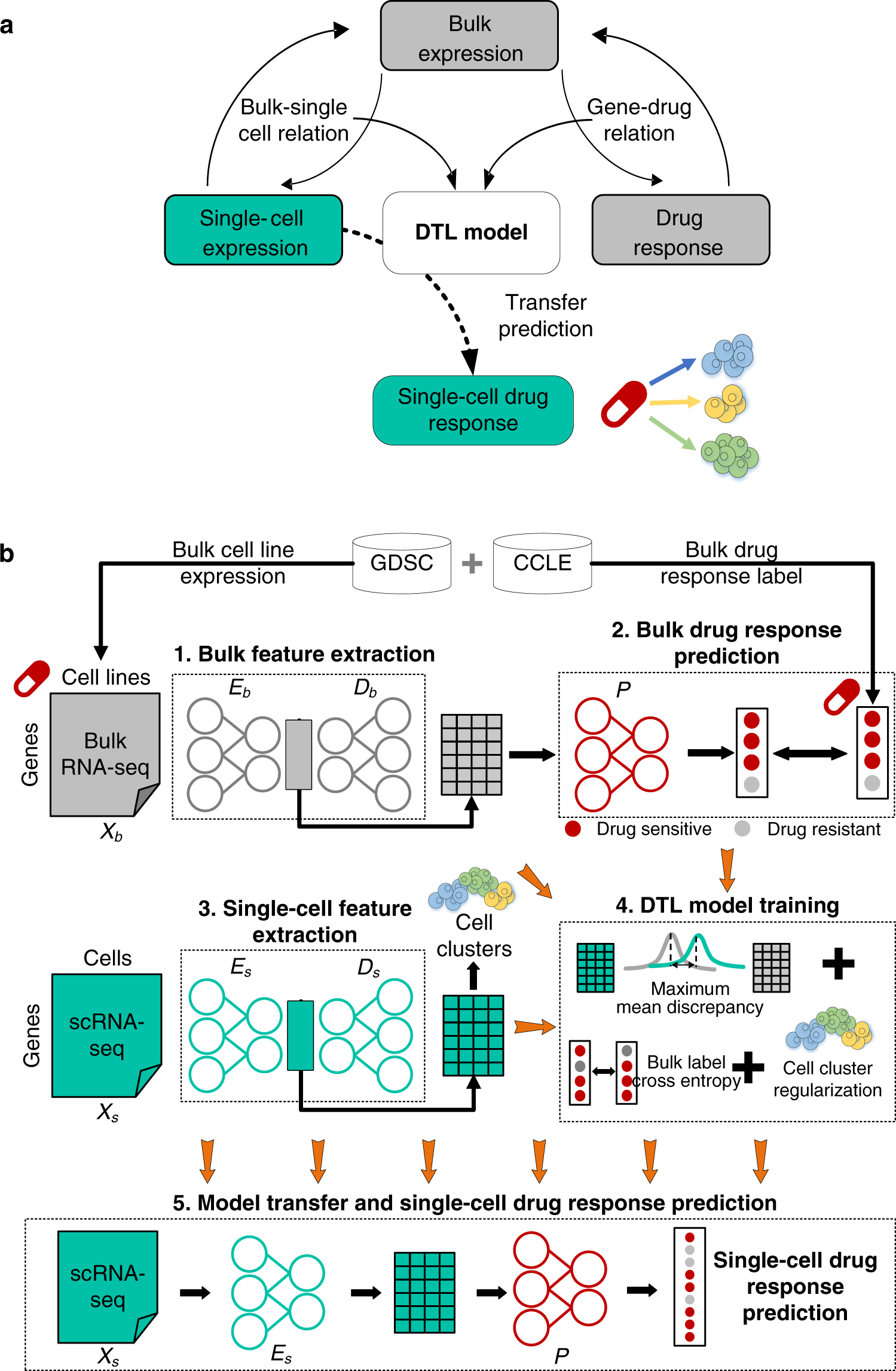
Deep transfer learning of cancer drug responses by integrating
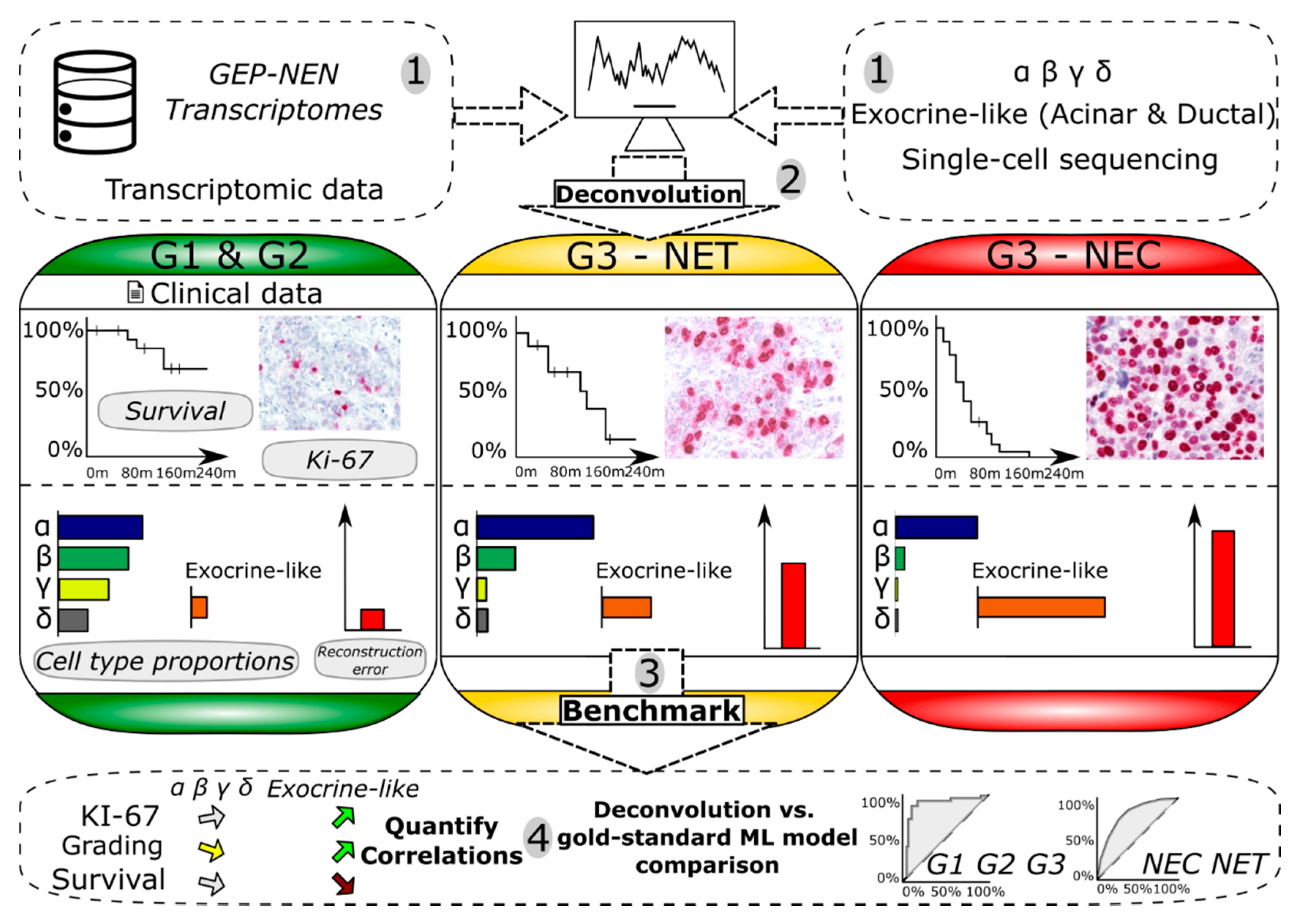
Cancers, Free Full-Text
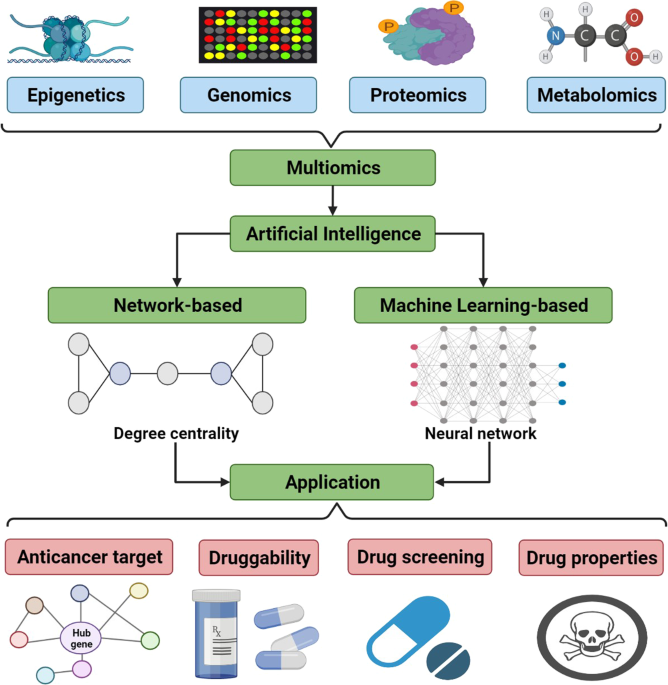
Artificial intelligence in cancer target identification and drug
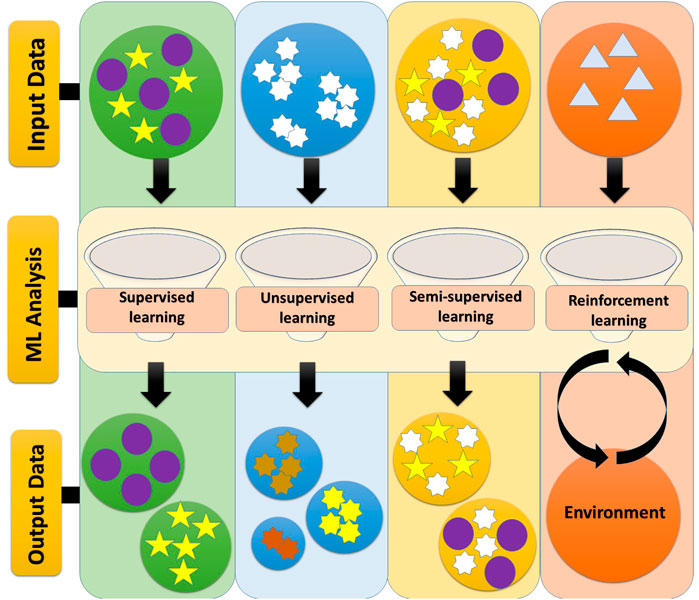
Frontiers Machine Learning: A New Prospect in Multi-Omics Data
Recomendado para você
-
Brain Test Level 367 answer/solution. #shorts #braintest29 março 2025
-
Brain Test Level 372 He wants big muscles in 202329 março 2025
-
Test your brain power on Zoom in August! - General News - News29 março 2025
-
Kunci Jawaban Brain Test Level 361 362 363 364 365 366 367 368 369 370: Saatnya Mencari Cuan - Tribunbengkulu.com29 março 2025
-
Lengkap Ada Video, Brain Test Level 367 Saatnya Mancari cuan! ✓29 março 2025
-
Test your brain Cold Spring Harbor Laboratory29 março 2025
-
Brain Test: Tricky Puzzles Answers for All Levels - Page 37 of 46 - Level Winner29 março 2025
-
Hereditary multiple exostoses with spinal cord compression.29 março 2025
-
Blinking a Book - Create Your Own Comic Strips Online with MakeBeliefsComix29 março 2025
-
What Is Serotonin?29 março 2025
você pode gostar
-
Conjunto do Flamengo Tradicional Modelo 2023-2024 Uniforme de29 março 2025
-
NoelStuder's Blog • Choosing the Right Chess Openings for Beginners – 3 Key Traits •29 março 2025
-
Zootopia 2' Release Date: Cast, & Everything We Know - Disney Plus Informer29 março 2025
-
Who is Radagon? Elden Ring lore29 março 2025
-
Giba Neles! (Em Portuguese do Brasil): 9788525060013: Giba: Libros29 março 2025
-
Just noticed this :_) Honkai: Star Rail29 março 2025
-
Candidates Tournament: Eight Compete for Spot in Chess Championship29 março 2025
-
Pokedex Hoenn for a Pokemon Card29 março 2025
-
Isekai Nonbiri Nouka - Final Trailer [Sub indo] - BiliBili29 março 2025
-
FCSB - FC Hermannstadt. Nu joacă palmaresul direct - Avancronică - LPF29 março 2025