AlphaDDA: strategies for adjusting the playing strength of a fully
Por um escritor misterioso
Last updated 03 abril 2025
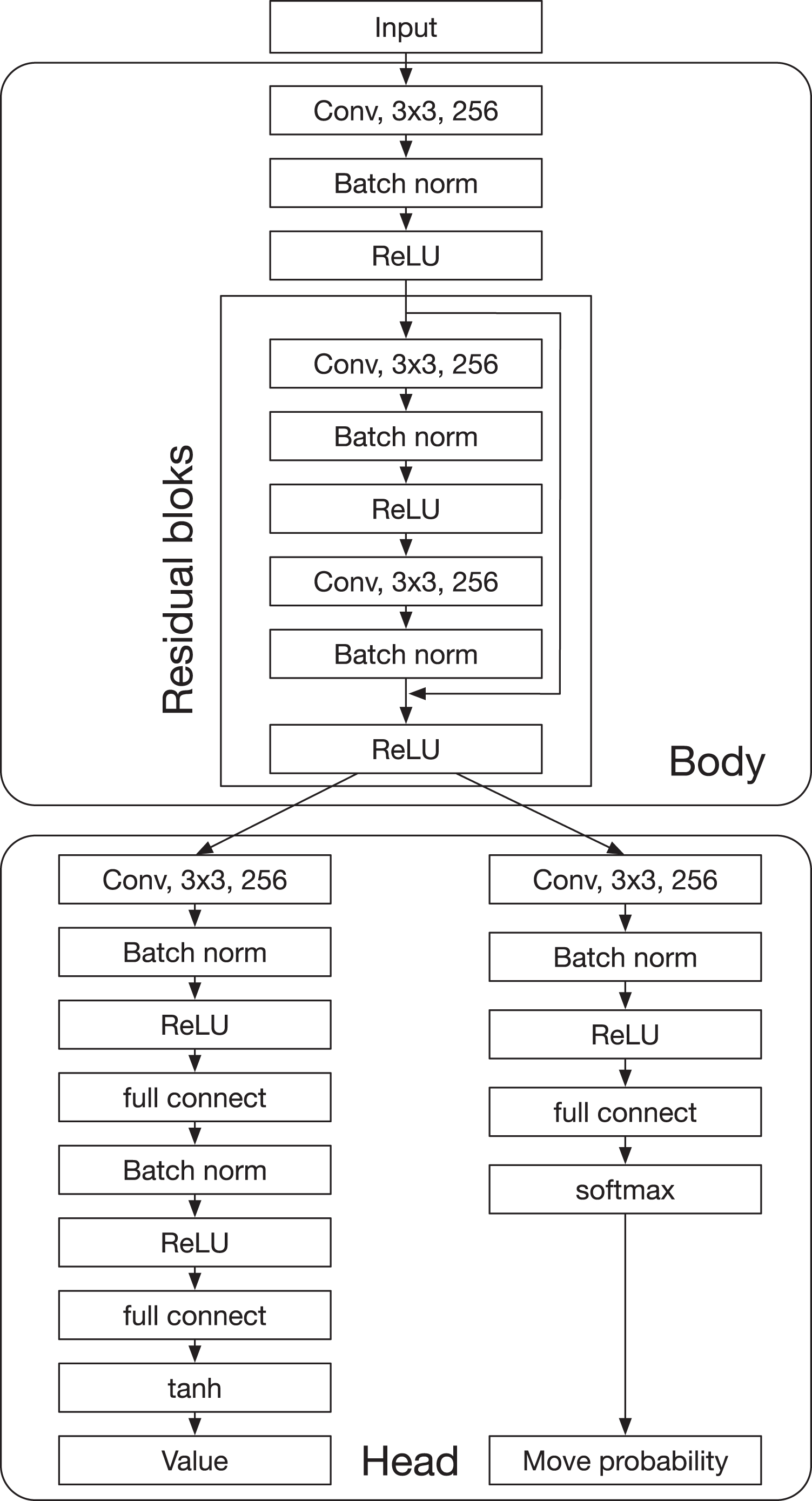
Artificial intelligence (AI) has achieved superhuman performance in board games such as Go, chess, and Othello (Reversi). In other words, the AI system surpasses the level of a strong human expert player in such games. In this context, it is difficult for a human player to enjoy playing the games with the AI. To keep human players entertained and immersed in a game, the AI is required to dynamically balance its skill with that of the human player. To address this issue, we propose AlphaDDA, an AlphaZero-based AI with dynamic difficulty adjustment (DDA). AlphaDDA consists of a deep neural network (DNN) and a Monte Carlo tree search, as in AlphaZero. AlphaDDA learns and plays a game the same way as AlphaZero, but can change its skills. AlphaDDA estimates the value of the game state from only the board state using the DNN. AlphaDDA changes a parameter dominantly controlling its skills according to the estimated value. Consequently, AlphaDDA adjusts its skills according to a game state. AlphaDDA can adjust its skill using only the state of a game without any prior knowledge regarding an opponent. In this study, AlphaDDA plays Connect4, Othello, and 6x6 Othello with other AI agents. Other AI agents are AlphaZero, Monte Carlo tree search, the minimax algorithm, and a random player. This study shows that AlphaDDA can balance its skill with that of the other AI agents, except for a random player. AlphaDDA can weaken itself according to the estimated value. However, AlphaDDA beats the random player because AlphaDDA is stronger than a random player even if AlphaDDA weakens itself to the limit. The DDA ability of AlphaDDA is based on an accurate estimation of the value from the state of a game. We believe that the AlphaDDA approach for DDA can be used for any game AI system if the DNN can accurately estimate the value of the game state and we know a parameter controlling the skills of the AI system.
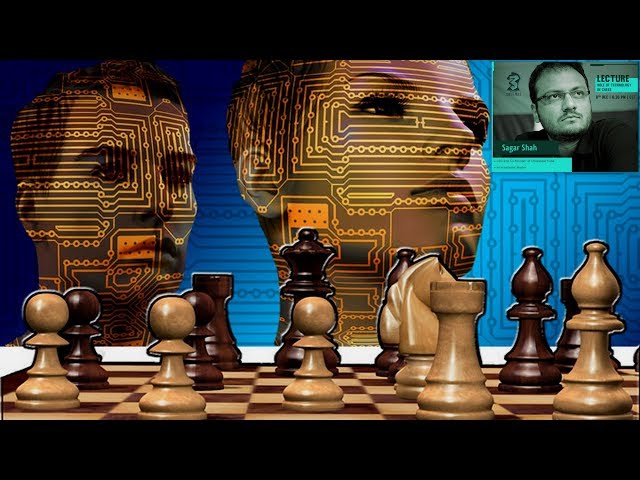
Game Changer: AlphaZero's Groundbreaking Chess Strategies and the Promise of AI

User learning curve Download Scientific Diagram

Slices of the (a) first (horizontal), (b) second (latteral) and (c)

Switching iterations for training on different games with different

Mastering the Card Game of Jaipur Through Zero-Knowledge Self-Play Reinforcement Learning and Action Masks

藤田 一寿 (Kazuhisa Fujita) - マイポータル - researchmap
Mastering the Card Game of Jaipur Through Zero-Knowledge Self-Play Reinforcement Learning and Action Masks
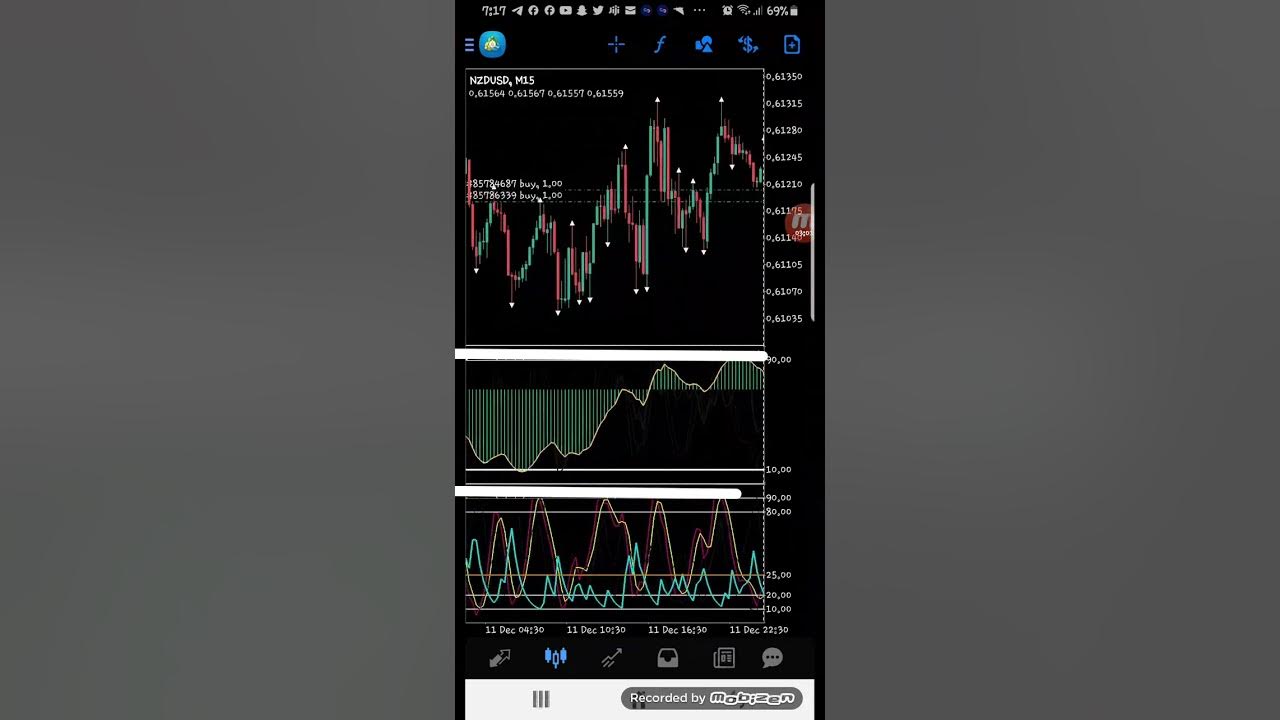
Alpha strategy 99.9 percent accuracy, works on all INSTRUMENTS #strategy #profit

Figure A2 Example of a game of MCTS2 (black) vs AlphaDDA1 (white) in
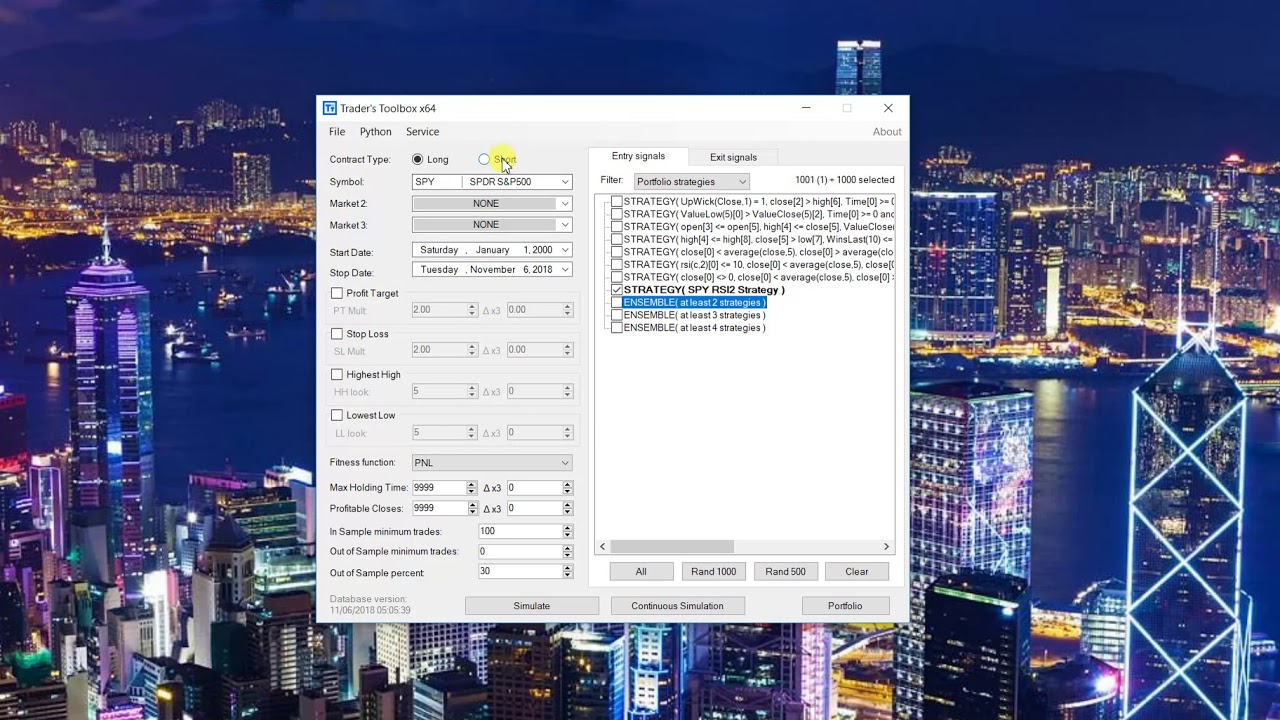
Build Alpha - Building Strategies Using Other Strategies

Build Alpha Reviews, Trading Reviews and Vendors

Elbow plot with the mean squared error as a function of the number of
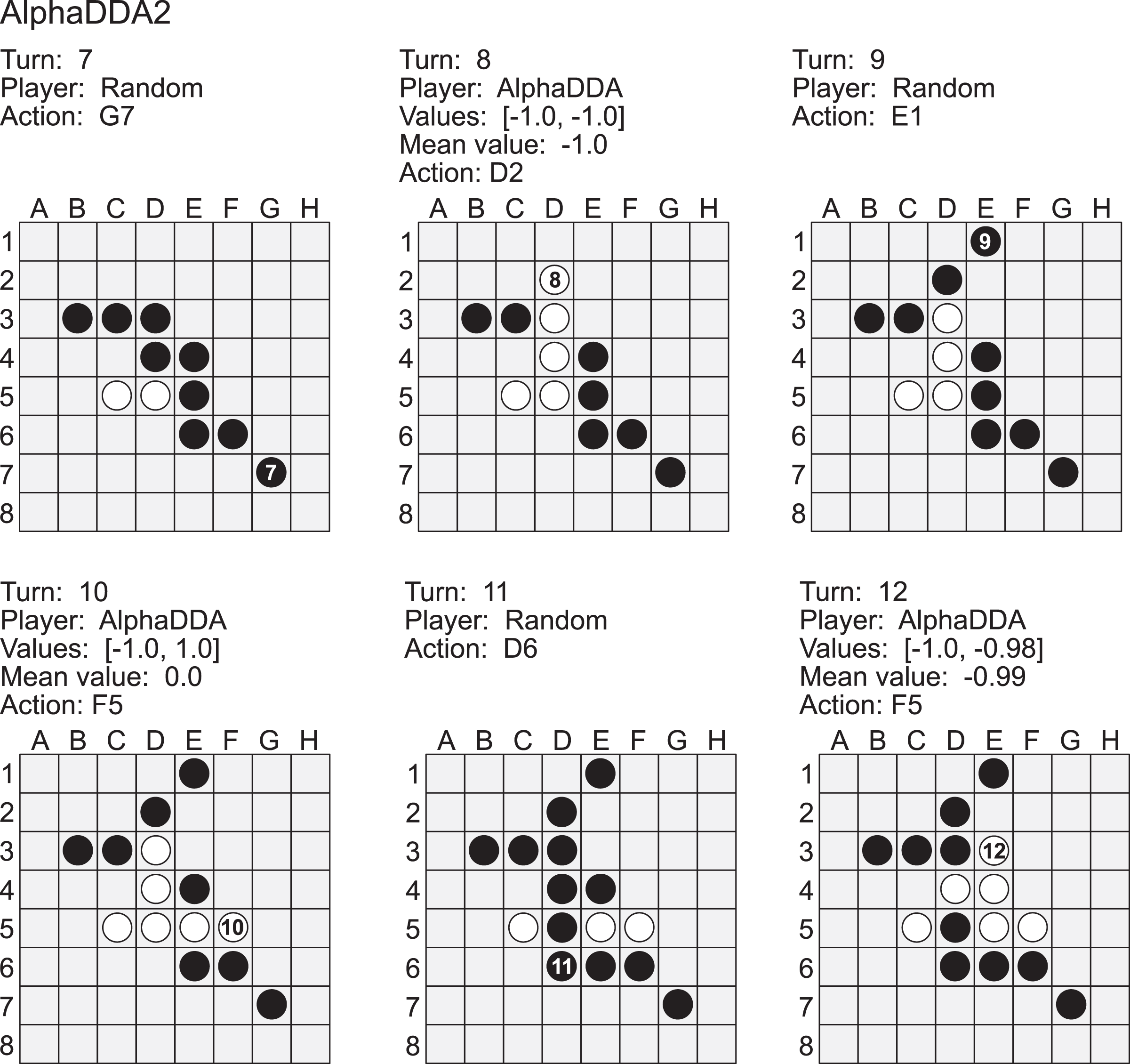
AlphaDDA: strategies for adjusting the playing strength of a fully trained AlphaZero system to a suitable human training partner [PeerJ]
Recomendado para você
-
Alphazero Chess Download PNG - Google-Keresés03 abril 2025
-
GitHub - PythonNut/alphazero-othello: An implementation of the AlphaZero algorithm for playing Othello (aka. Reversi)03 abril 2025
-
Even AlphaZero Found This Game Hard03 abril 2025
-
AlphaZero_Connect4/README.md at master · plkmo/AlphaZero_Connect403 abril 2025
-
GitHub - peldszus/alpha-zero-general-lib: An implementation of the03 abril 2025
-
alphazero · GitHub Topics · GitHub03 abril 2025
-
Montreal.AI - TensorFlow.js Implementation of DeepMind's AlphaZero03 abril 2025
-
Time manager Alphazero - Leela Chess Zero03 abril 2025
-
redis - golang Package Health Analysis03 abril 2025
-
Acquisition of Chess Knowledge in AlphaZero03 abril 2025
você pode gostar
-
BOKURA WA MINNA KAWAISOU ORIGINAL SOUNDTRACK Between the Notes03 abril 2025
-
JogaBets App Moçambique 2023 – Baixar Joga Bets para Android (.apk03 abril 2025
-
Dama da Noite (Deusas da Morte) eBook : Reys, Ellen: : Loja Kindle03 abril 2025
-
Jogo de Panelas Tramontina Vermont em Alumínio com Revestimento03 abril 2025
-
Slides 02 - Jogos Eletrônicos, PDF03 abril 2025
-
Tua Tagovailoa contract: What could Dolphins be looking at?03 abril 2025
-
Factorio is coming to Nintendo Switch™03 abril 2025
-
UFL: novo jogo de futebol online e gratuito quer brigar com FIFA e03 abril 2025
-
The Last Summoner Awakening - Watch on Crunchyroll03 abril 2025
-
codigos secretos netflix de anime|Pesquisa do TikTok03 abril 2025