Policy or Value ? Loss Function and Playing Strength in AlphaZero-like Self-play
Por um escritor misterioso
Last updated 01 abril 2025
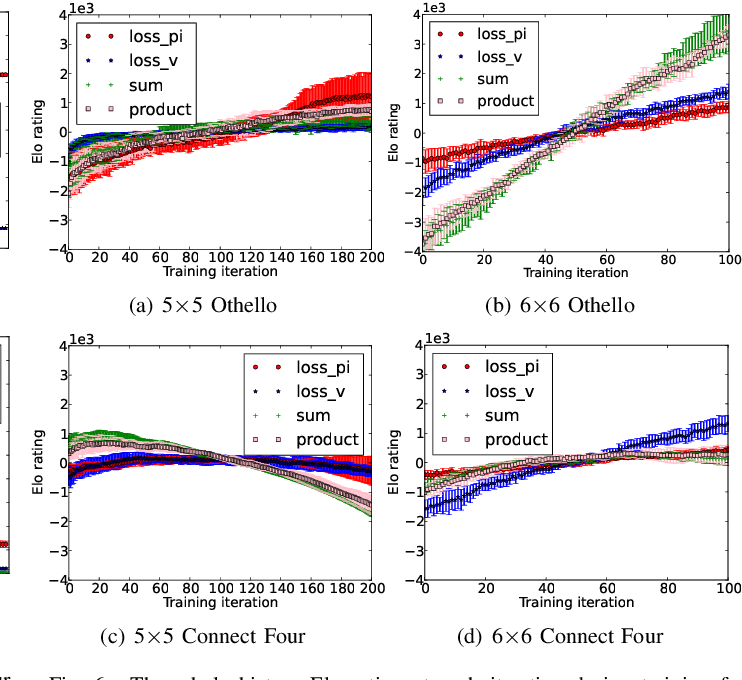
Results indicate that, at least for relatively simple games such as 6x6 Othello and Connect Four, optimizing the sum, as AlphaZero does, performs consistently worse than other objectives, in particular by optimizing only the value loss. Recently, AlphaZero has achieved outstanding performance in playing Go, Chess, and Shogi. Players in AlphaZero consist of a combination of Monte Carlo Tree Search and a Deep Q-network, that is trained using self-play. The unified Deep Q-network has a policy-head and a value-head. In AlphaZero, during training, the optimization minimizes the sum of the policy loss and the value loss. However, it is not clear if and under which circumstances other formulations of the objective function are better. Therefore, in this paper, we perform experiments with combinations of these two optimization targets. Self-play is a computationally intensive method. By using small games, we are able to perform multiple test cases. We use a light-weight open source reimplementation of AlphaZero on two different games. We investigate optimizing the two targets independently, and also try different combinations (sum and product). Our results indicate that, at least for relatively simple games such as 6x6 Othello and Connect Four, optimizing the sum, as AlphaZero does, performs consistently worse than other objectives, in particular by optimizing only the value loss. Moreover, we find that care must be taken in computing the playing strength. Tournament Elo ratings differ from training Elo ratings—training Elo ratings, though cheap to compute and frequently reported, can be misleading and may lead to bias. It is currently not clear how these results transfer to more complex games and if there is a phase transition between our setting and the AlphaZero application to Go where the sum is seemingly the better choice.

Strength and accuracy of policy and value networks. a Plot showing
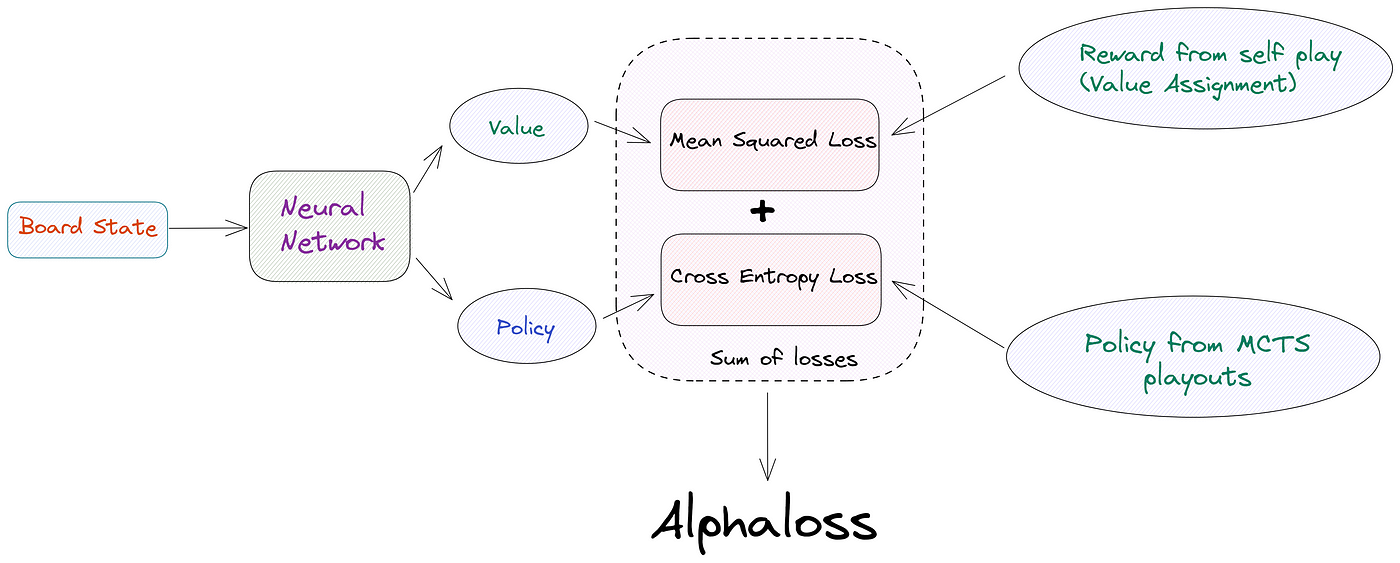
AlphaZero from scratch in PyTorch for the game of Chain Reaction

LightZero: A Unified Benchmark for Monte Carlo Tree Search in
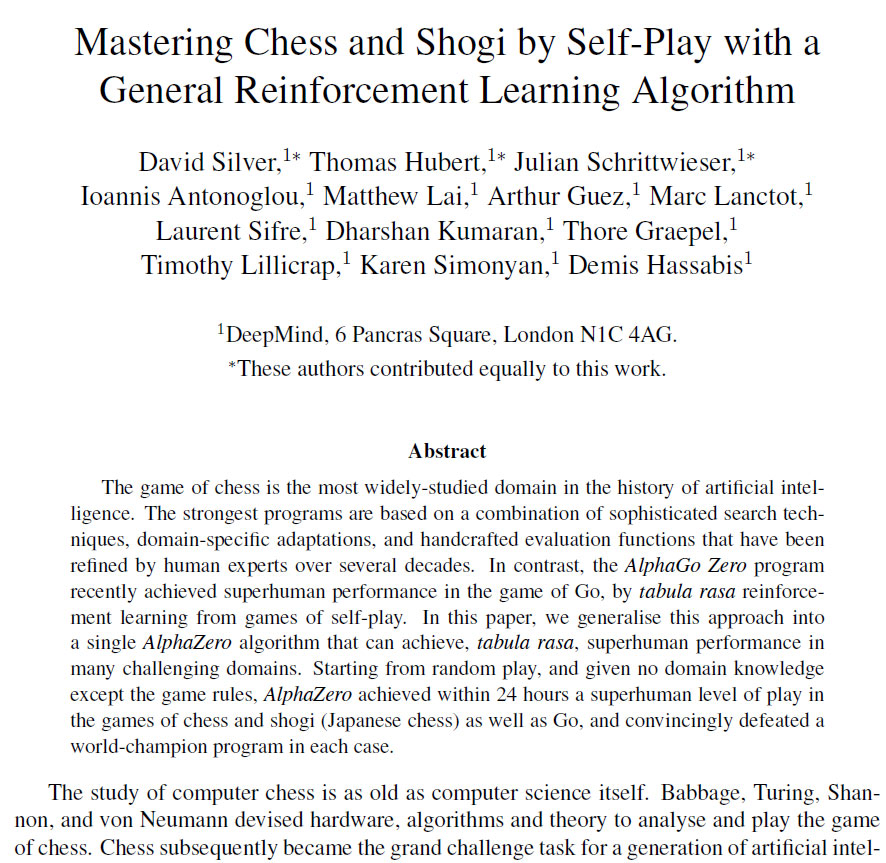
The future is here – AlphaZero learns chess

AlphaZero
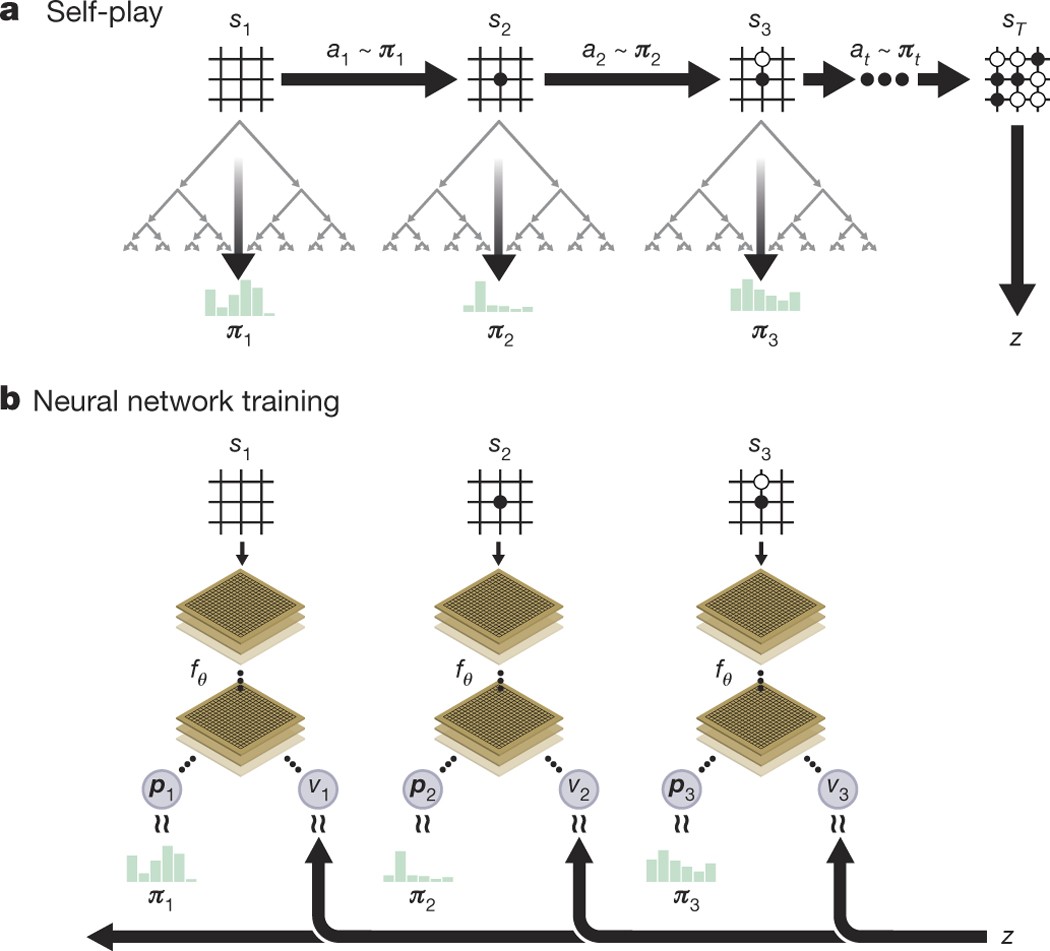
Mastering the game of Go without human knowledge
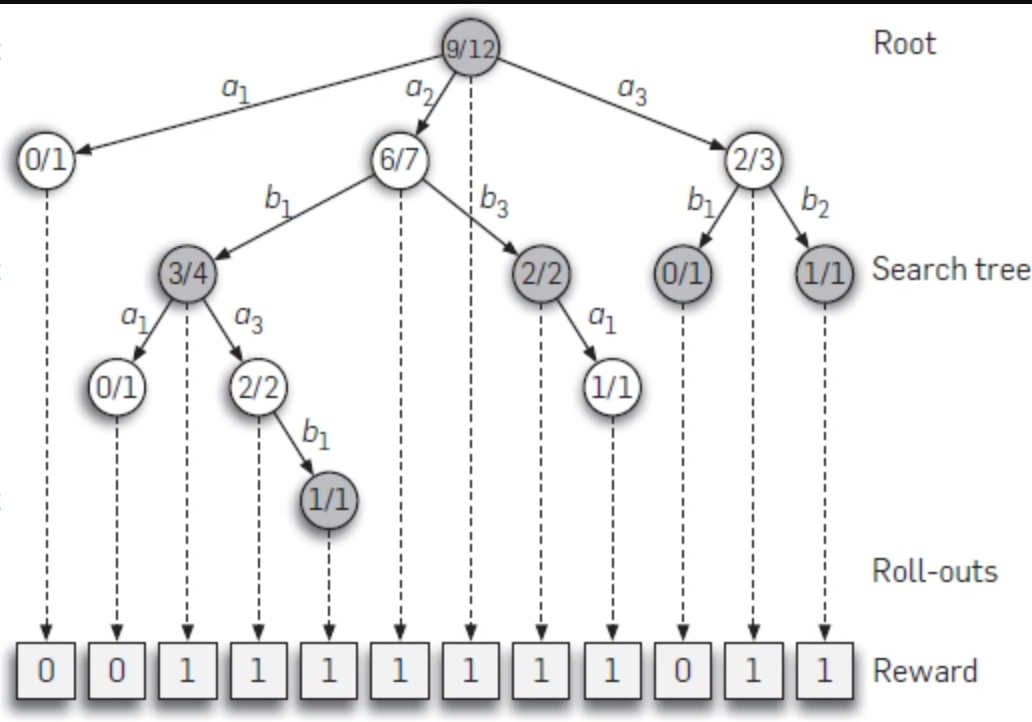
AlphaZero Explained · On AI
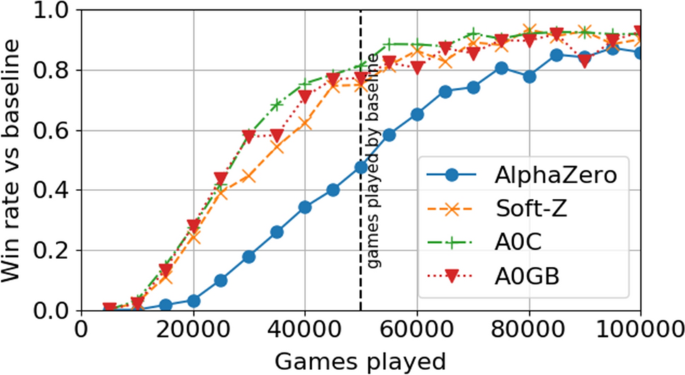
Value targets in off-policy AlphaZero: a new greedy backup
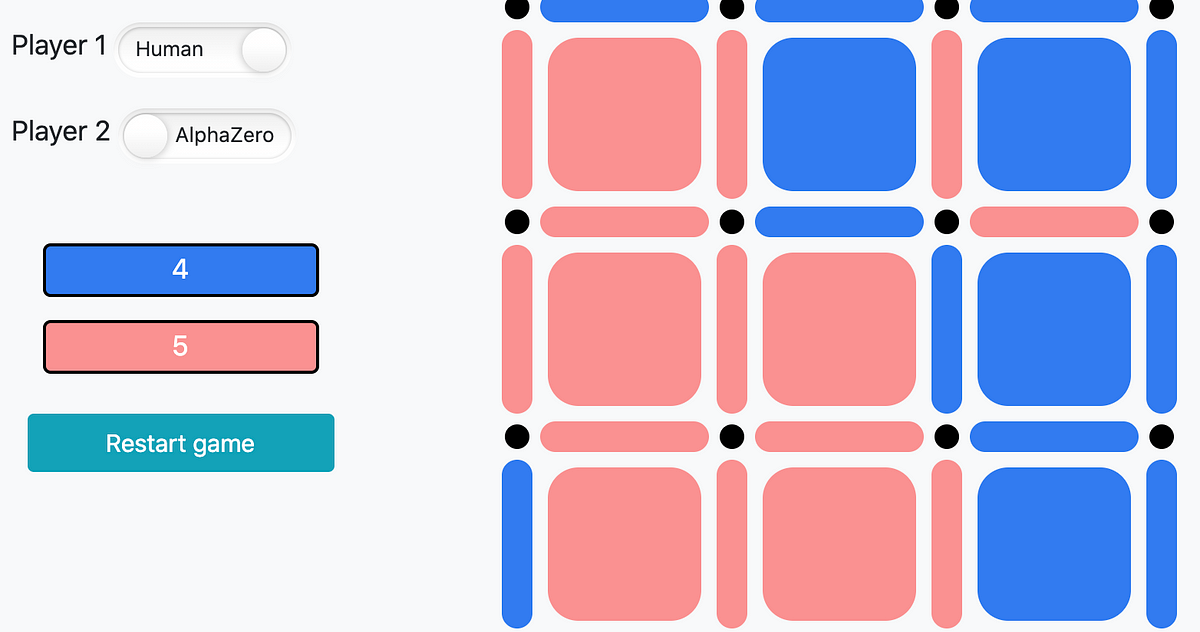
AlphaZero, a novel Reinforcement Learning Algorithm, in JavaScript

Why Artificial Intelligence Like AlphaZero Has Trouble With the
Recomendado para você
-
Leela Chess Zero: AlphaZero for the PC01 abril 2025
-
Google's AlphaZero Destroys Stockfish In 100-Game Match01 abril 2025
-
Did AlphaZero also have to learn that each piece has a value? - Chess Stack Exchange01 abril 2025
-
ELO Ratings Benchmark (Game of Shogi)01 abril 2025
-
Prevelo Alpha Zero Review: Why It Earns our Highest Rating01 abril 2025
-
Alphazero Performed 4000 Elo Game Against Magnus Carlsen, Alphazero vs Magnus Carlsen01 abril 2025
-
AlphaGo Zero Explained01 abril 2025
-
AlphaZero: Four Hours to World Class from a Standing Start - Breakfast Bytes - Cadence Blogs - Cadence Community01 abril 2025
-
Training AlphaZero for 700,000 steps. Elo ratings were computed from01 abril 2025
-
AlphaZero paper peer-reviewed is available · Issue #2069 · leela-zero/leela- zero · GitHub01 abril 2025
você pode gostar
-
Pin on Sanepo Updates01 abril 2025
-
Penalty Shooters 2 Play Now Online for Free01 abril 2025
-
A Roblox Guest's First Selfie by KellyKey on Newgrounds01 abril 2025
-
10 Animes Para Se Ver Dublado - Página 7 de 11 - Anime United01 abril 2025
-
Jogo De Cozinha Oxford Girassóis 7 Peças Com Brinde Surpresa01 abril 2025
-
Como desenhar um cavalo FACIL passo a passo para crianças e iniciantes 101 abril 2025
-
Kollywood's biggest stars to clash in the next four months - Bollywood News01 abril 2025
-
Amber Ardolino WIll Join the Cast of A BEAUTIFUL NOISE as 'Marcia Murphy' Next Year01 abril 2025
-
hle: Not enough space, negative space needed · Issue #7084 · RPCS3/rpcs3 · GitHub01 abril 2025
-
ADF-11F Ace Combat 3 Pack Mod - Ace Combat 7: Skies01 abril 2025