Efficient and Accurate Candidate Generation for Grasp Pose
Por um escritor misterioso
Last updated 24 novembro 2024

Recently, a number of grasp detection methods have been proposed that can be used to localize robotic grasp configurations directly from sensor data without estimating object pose. The underlying idea is to treat grasp perception analogously to object detection in computer vision. These methods take as input a noisy and partially occluded RGBD image or point cloud and produce as output pose estimates of viable grasps, without assuming a known CAD model of the object. Although these methods generalize grasp knowledge to new objects well, they have not yet been demonstrated to be reliable enough for wide use. Many grasp detection methods achieve grasp success rates (grasp successes as a fraction of the total number of grasp attempts) between 75% and 95% for novel objects presented in isolation or in light clutter. Not only are these success rates too low for practical grasping applications, but the light clutter scenarios that are evaluated often do not reflect the realities of real world grasping. This paper proposes a number of innovations that together result in a significant improvement in grasp detection performance. The specific improvement in performance due to each of our contributions is quantitatively measured either in simulation or on robotic hardware. Ultimately, we report a series of robotic experiments that average a 93% end-to-end grasp success rate for novel objects presented in dense clutter.
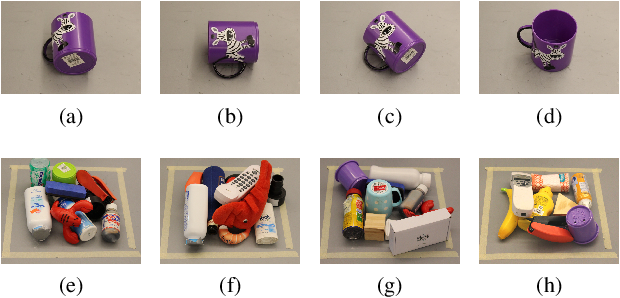
PDF] Efficient and Accurate Candidate Generation for Grasp Pose

Evaluation on the GraspNet dataset

Grasp Pose Detection in Point Clouds - Andreas ten Pas, Marcus
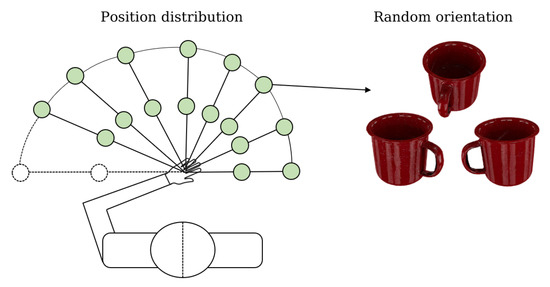
Biomimetics, Free Full-Text

A grasps-generation-and-selection convolutional neural network for

Grasp Pose Detection in Point Clouds - Andreas ten Pas, Marcus

Efficient and Accurate Candidate Generation for Grasp Pose
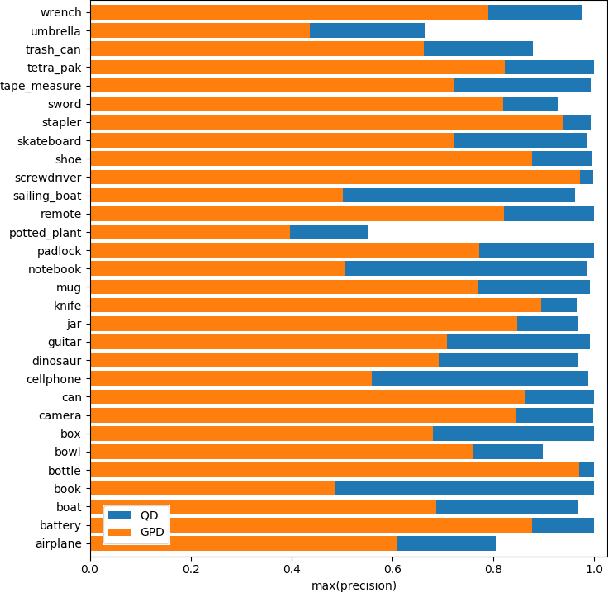
PDF] Efficient and Accurate Candidate Generation for Grasp Pose
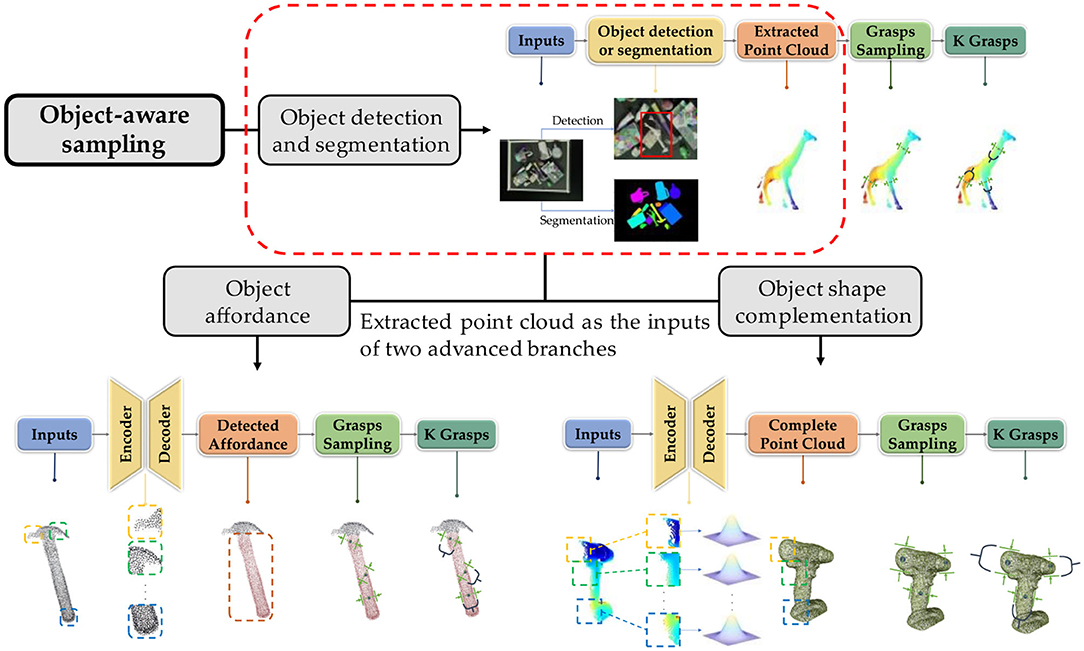
Frontiers Robotics Dexterous Grasping: The Methods Based on
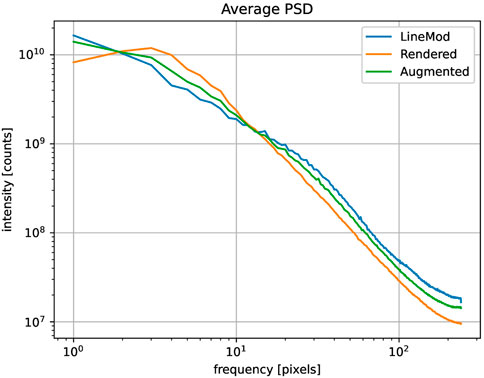
Frontiers 6IMPOSE: bridging the reality gap in 6D pose
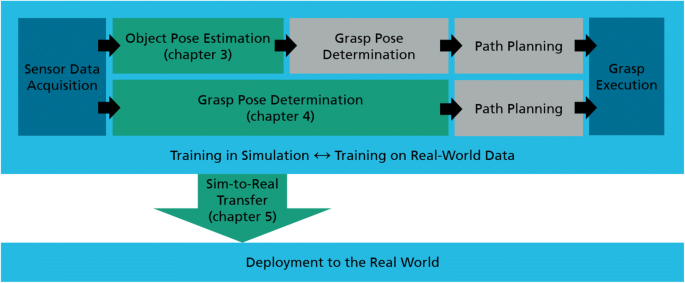
A Survey on Learning-Based Robotic Grasping

Grasp Pose Detection in Point Clouds - Andreas ten Pas, Marcus
Recomendado para você
-
Candidate-SE - Secretaria Nacional de Organização do PT24 novembro 2024
-
La France au Mozambique on X: 🎓📚🇫🇷⚠️Candidate-se a uma24 novembro 2024
-
Se Candidate Mulher24 novembro 2024
-
Efeito Mais no LinkedIn: ➡ CANDIDATE-SE! ✓ Oportunidade de24 novembro 2024
-
Candidate-se a um emprego. empregadores e vagas, envie seu cv24 novembro 2024
-
Concept of ferrielectricity and candidate material BaFe 2 Se 3 . a24 novembro 2024
-
Empregos na S&C Electric Company24 novembro 2024
-
Eleição para o Conselho Tutelar: servidor, candidate-se - SISMUC24 novembro 2024
-
Colocamos ao menos uma mulher por dia no mercado de trabalho24 novembro 2024
-
Arquitetura e Engenharia: Candidate-se em vagas da semana de24 novembro 2024
você pode gostar
-
SCP-008-J - Geoff The SCP Foundation Database24 novembro 2024
-
Does Dead Island 2 have Crossplay and Cross Save? Find Now!24 novembro 2024
-
Metacritic - The Best-Reviewed PS5 Games of All-Time: #6 - Horizon Forbidden West [89]24 novembro 2024
-
Blast from the Past: Star Wars Rogue Squadron II: Rogue Leader (GC) - Nintendo Blast24 novembro 2024
-
Nero Piezas de ajedrez de alto polímero extra pesadas con reinas adicionales- Piezas solamente - Sin tablero - Rey de 4.25 in : Juguetes y Juegos24 novembro 2024
-
Valorant Boost - Net Wins Boost24 novembro 2024
-
Percy Jackson and the Olympians Is This Week's Black TV Pick24 novembro 2024
-
Resultado do jogo Genoa x AS Roma hoje, 28/9: veja o placar e estatísticas da partida - Jogada - Diário do Nordeste24 novembro 2024
-
NieR: Automata Anime's Rocky Production Hit With More Delays24 novembro 2024
-
Apresentação da versão digital do jogo educacional Desafios com24 novembro 2024